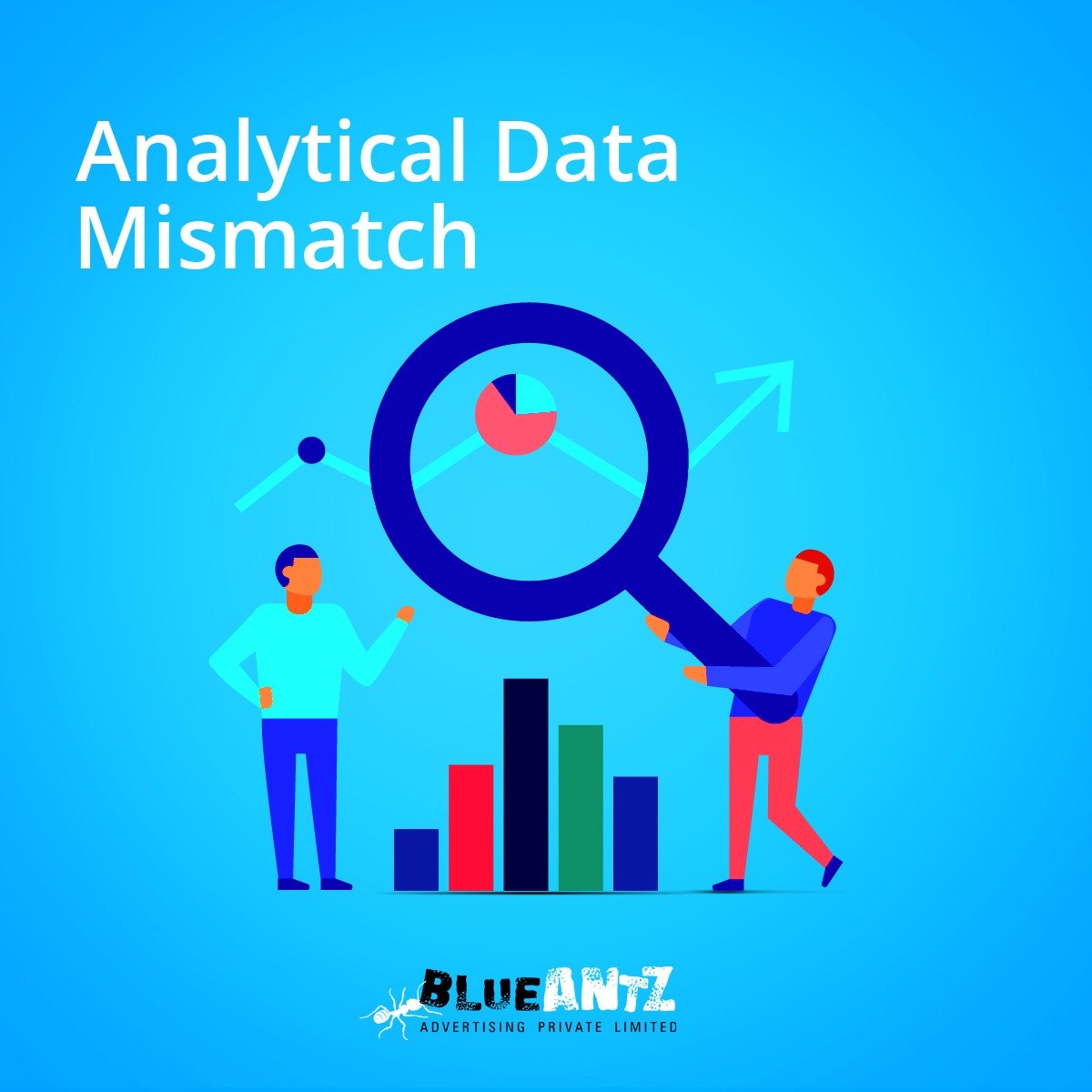
Analytical Data & Client’s End Mismatch- How to Overcome
Analytical data is cumulative data that benefits in decision making or carrying out researches like Google Analytics, Facebook Analytics, Twitter Analytics, and LinkedIn Analytics etc. Digital marketers collect data from these tools to perform analytics and plan the next steps accordingly as well as make scaling reports as well.
These historical data are stored in read-only databases and are optimized for data analysis; these are basically raw data, helping in identifying patterns, detecting important and useful information to draw a comprehensive conclusion. Data analysis plays a vital role in operating business more efficiently in today’s world.
Types of Analytical Data
There are mainly four types of analytics
Descriptive Analytical Data
Descriptive data analysis is basically summing up data in a statistical way that helps in identifying patterns with the help of historical data.
For example – when a person is conducting a live session on any platform to sell their products, the descriptive analysis will provide the data on how many people participated and also how many times a particular person posted during these live sessions.
Historical data can be found with the help of data aggregation or the data mining process.
Descriptive analysis can be used to track different course enrolments and course compliance rates. They can also sum up the number of times a learner posts on a particular discussion board, track assignments etc.
Predictive Analysis
Predictive analysis is data extraction from the existing set of data in order to identify the patterns and trends. This tool helps in predicting the outcome and provides the companies with a comprehensive forecast of the future behaviour of buyers.
The data extracted from predictive data, turns raw data into informative insight, therefore help marketers to understand customer behaviour and generate effective marketing approaches and offers.
Predictive analysis can be used for designing promotions based on the interest of the customer, creating offers, combustions of right and useful products for the target audience and a lot more.
For example- when we check or make any purchase online, after making the purchase we get to see ads of the same kind of item with offers and discounted prices. This is done with the help of predictive analysis.
Exploratory Analysis
Exploratory analysis is a critical process via which the data is initially investigated to discover patterns, making the data comprehensible by summing up their main characteristics. This is the first step for data analysis; it is extremely useful to the analyst.
Inferential Analysis
This is a data collecting process, where the data is collected from the mass and make predictions. The inferential analysis is done on calculations like Linear Regression Analysis, Logistic Regression Analysis, analysis of Variance (ANOVA), Analysis of Covariance (ANCOVA), Statistical Significance (T-Test) and Correlation Analysis.
Mismatch from Clients’ End
The data analysis process starts from data collection, data cleaning, manipulation of data, analysing data and data visualization. So we always set benchmarks for our clients every financial year, convey the same to the client and set our targets accordingly.
But it has been seen or experienced that while making monthly reports for our clients there is a mismatch in the goals section of the analytics where we track the footfalls. For example, when we create a page with a validated form after a user fills up the form, there comes a thank you page. This is where we set the goal in analytics using the URL of the thank you page and also set UTM to track the exact data. So we get the leads once people click the submit button through mails as well.
It can happen that one single person clicks and fills up on the same several times. This can cause the difference in the analytical data and the lead generation as analytics treats them several leads and increases goals accordingly but on the client’s end it’s treated as one lead.
The same happens for ranking positions. Sometimes console-derived analytical data in Google Analytics show random results when the actual ranking positions for search queries in Google SERP showing the different results. So marketers double-check these manually.
This can be further differentiated between paid and organic, therefore we need to split the analysis part by part and come up with the actual data and the real number of conversions. Problem with not set (not the not provided data) is also a regular phenomenon. Marketers do the next step analysis for the details.